Statistics Seminar: Guanyang Wang
Virtual Event
Speaker: Guanyang Wang, Rutgers
Title: Unbiased optimal stopping via the MUSE
Abstract: We propose a new unbiased estimator for estimating the utility of the optimal stopping problem. The MUSE, short for`Multilevel Unbiased Stopping Estimator', constructs the unbiased Multilevel Monte Carlo (MLMC) estimator at every stage of the optimal stopping problem in a backward recursive way. In contrast to traditional sequential methods, the MUSE can be implemented in parallel when multiple processors are available. We prove the MUSE has finite variance, finite computational complexity, and achieves *$\varepsilon$-accuracy with $O(1/\varepsilon^2)$ computational cost under mild conditions. We demonstrate MUSE empirically in several numerical examples, including an option pricing problem with high-dimensional inputs, which illustrates the use of the MUSE on computer clusters. This is joint work with Zhengqing Zhou, Jose Blanchet and Peter Glynn.
*$ symbols are typed in the latex math environment
Bio: Guanyang Wang is an assistant professor at Rutgers. He did his Ph.D. at Stanford math working with Professor Persi Diaconis. His research interests include Monte Carlo methods especially Markov chain Monte Carlo, probability and Bayesian statistics.
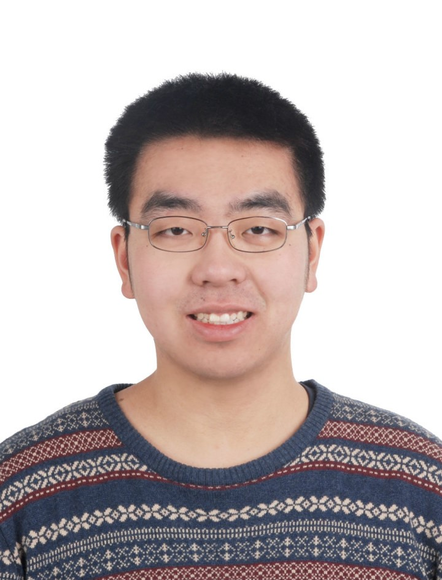